Digital twin accelerates solar research
Interdisciplinary team uses AI for the development of organic photovoltaics
Artificial intelligence is expected to accelerate the search for the perfect material for solar panels by a factor of ten. An interdisciplinary team from FAU is working on this. Researchers from engineering including materials science, chemistry, and computer science aim to implement a digital twin that better characterizes material combinations and leads high-throughput experiments to success more quickly. The concept was introduced in the renowned journal Joule.
Already today, up to 60 percent of electricity in Germany is generated by photovoltaics on sunny days. This is good for the climate but consumes a lot of space. Not least for this reason, organic photovoltaics based on carbon compounds offer an important perspective: “Organic solar modules can be flexible and also transparent,” explains Prof. Dr. Christoph J. Brabec, Professor of Materials in Electronics and Energy Technology at FAU and Director at the Helmholtz Institute Erlangen-Nürnberg (HI ERN). “They can be integrated into windows and facades, used in interiors, or deployed in fields as coverings under which plants can grow.” However, significant progress is still needed for successful commercialization, especially since requirements for performance, durability, and recyclability sometimes compete with each other.
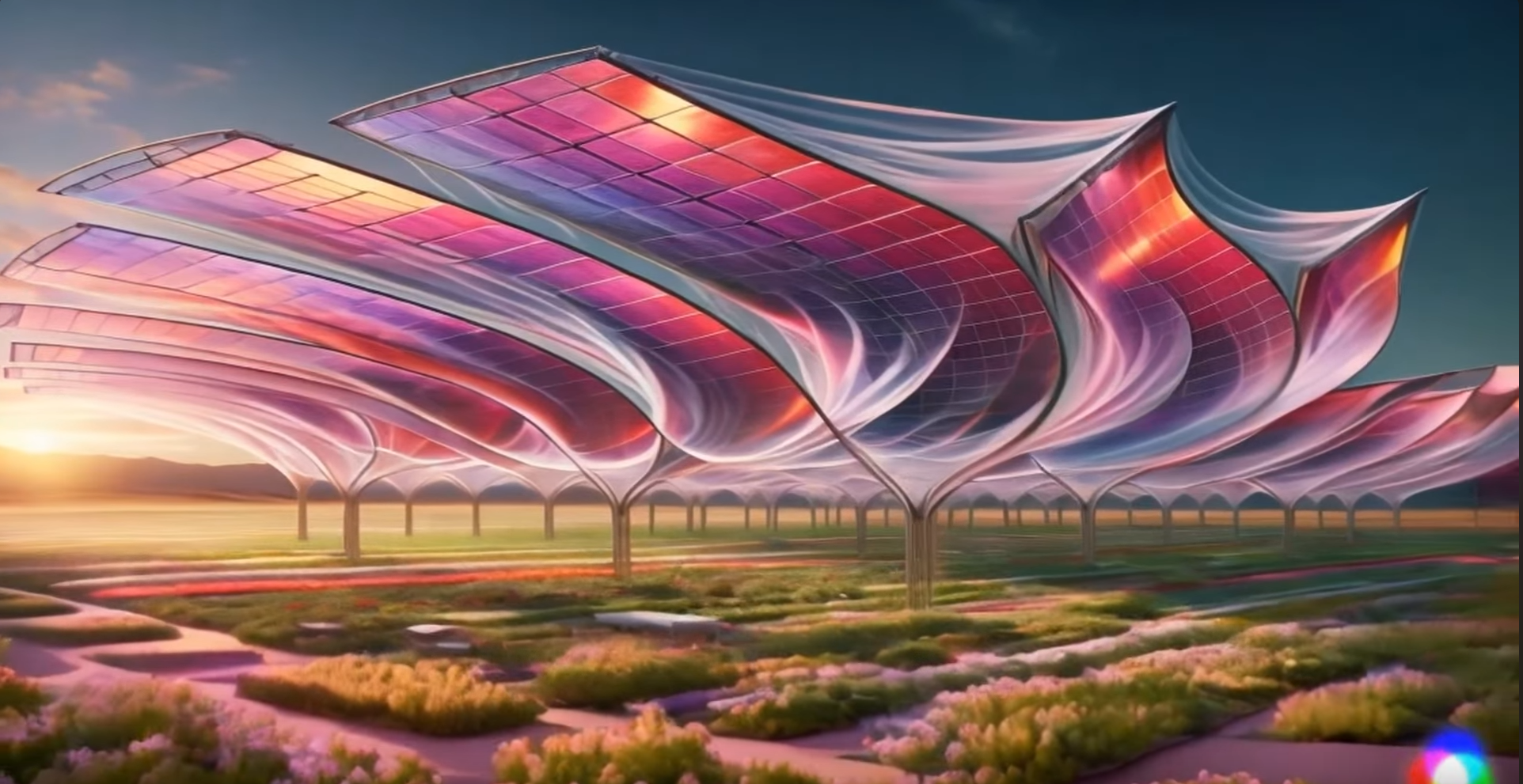
immensely versatile.
Optimizing microstructure with AI
The performance of materials crucially depends on their microstructure. While it can be simulated in computer programs, practical characterization is laborious and destructive, which delays breakthroughs. Therefore, the research team at the FAU Solar Profile Center is taking a new, unique approach in photovoltaic research. The experts from materials science, engineering, chemistry, and computer science are implementing a so-called digital twin – an AI-based replica of the physical analysis processes. “The AI provides us with decision support in real-time, even when the information is sparse and indirect,” says Dr. Larry Lüer, group leader for Photovoltaic Applications and Machine Learning at FAU.
Model calculations for identifying relationships between structure and function are often very time-consuming, which slows down knowledge acquisition. Machine learning, on the other hand, is able to identify the decisive structural patterns independently: Which components and connections are dead ends and which are game changers? “AI helps us to find the common descriptor, that is, the characteristic property of all the different structures tested,” explains Lüer. “It learns from mistakes and dead ends and can make valuable predictions for promising material combinations.” As knowledge of the crucial descriptors greatly accelerates physical modeling, experts in solid-state calculations and mathematics are also involved in the consortium.
Minutes instead of days
The researchers expect significantly faster breakthroughs in the development of high-performance photovoltaic modules from the use of the digital twin. “It is difficult to estimate just how strong this acceleration is,” says Christoph Brabec. “Some processes, such as the characterization of the microstructure, could take only minutes instead of days.” Although this cannot be directly transferred to the discovery of new materials and the market readiness of the modules, the team still aims to reduce the development time by a factor of ten overall. A hopeful message for Germany’s ambitious goals on the path to climate neutrality.
Further information:
DOI: 10.1016/j.joule.2023.12.010 “A digital twin to overcome long-time challenges in photovoltaics”
Dr. Larry Lüer
Department of Materials Science
Phone: +49 9131 85-27472