A two-way street
The human brain is a hotbed of activity, with billions of neurons tirelessly communicating via trillions of synapses. One of the major benefits of this complexity is our ability to speak and understand language. Patrick Krauss is using artificial intelligence (AI) to research the neural basis of this ability.
It may well have been an amusing story, but listening to the audiobook wasn’t all fun for the participants. Not only did they have to answer comprehension questions every four minutes, they also had to keep their eyes on a cross on the screen whilst listening, at the same time as ignoring the cumbersome magnetoencephalography fitted over the top half of their head to measure their brainwaves. However, it was all for the benefit of science.
‘Using language to communicate is perhaps the most complex cognitive ability of the human race; perception, attention, learning and memory are just some of the factors involved,’ explains the principal investigator Dr. Patrick Krauss from the Cognitive Computational Neuroscience Group at FAU. Krauss is using heavy machinery in an attempt to pin down this ability. Along with magnetoencephalography (MEG), the researchers are using functional magnetic resonance imaging (fMRT) and electroencephalography (EEG), as well as very fine electrodes implanted directly into the brain. They all allow the researchers to take an (albeit indirect) look into the brain at work, showing how active the individual areas in the brain are when the test person is, for example, listening or speaking.
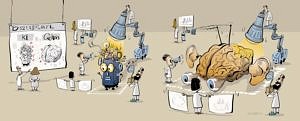
Krauss is using methods taken from the toolbox for research into artificial intelligence to analyse and visualise the collected data. This is not the only time he has turned to artificial intelligence for help. ‘If we want to understand how language is implemented in the brain, we need to build models of the processes in the brain and these models are based on AI,’ explains Krauss. ‘We can then experiment with these models and compare the results to data from behavioural experiments. Based on these comparisons, we can fine-tune the accuracy of the models.’
Krauss has a doctoral degree in physics, is currently working at the Chair for Experimental Otolaryngology on postdoctoral research in linguistics and regards himself as cognitive scientist. ‘An entirely new discipline is currently emerging between AI, mathematical modelling, neurosciences and linguistics: cognitive computational neuroscience,’ says Krauss. At the heart of this research area is the conviction that artificial intelligence and neurosciences can learn a lot from each other. Research into artificial intelligence never just intended to build machines to relieve us of tedious tasks. From the outset, the aim was also to design and test theories about natural intelligence. ‘The physicist Richard Feynman once said “what I cannot create, I do not understand”,’ continues Krauss. ‘That inspired me. Neurosciences and research into artificial intelligence are two sides of one coin.’
The brain has been compared to a number of technologies in an attempt to understand how it works: to the water pipes in an irrigation system which transport the water to wherever it is currently needed, to a church organ with its complicated array of pipes and to a telephone switchboard which connects incoming messages to the correct recipient. None of these comparisons have proven to be entirely fitting. Why should the computer and its algorithms be any different? It has been known for quite a while that the artificial neural networks which are involved in modelling cognitive abilities in the computer are only vaguely inspired by the way the brain works. They cannot compete with the complexity of their natural counterpart.
Krauss does not share these misgivings. As he sees it, ‘AI aims to attain cognition and behaviour at a human level, and the neurosciences aim to understand cognition and behaviour – the one complements the other.’ ‘In order to act as a model, the algorithmic systems do not necessarily need to be particularly similar to the human brain. If you copy the brain too exactly, this can lead to confusion. If you want to understand the law of gravity, you don’t start by trying to understand all the ins and outs of how a leaf falls from a tree and slowly makes its way to the ground.’
The important thing, according to Krauss, is to find the right level of abstraction: not too detailed, but still detailed enough. If the researchers compare a simulation of the processes in the brain with the processes in a real brain, they will ideally find common principles for intelligence which apply to humans and machines alike: ‘It has been shown that processing units in artificial neural networks which have been trained to recognise images specialise in abstract notions such as numbers, in other words they are only triggered when a certain number of objects can be seen, similarly to the human brain. It’s quite eye-opening,’ says Krauss.
One problem with this strategy, however, is that it is not much easier to decipher how neural networks work to solve problems than it is to unveil the workings of the human brain. Neural networks are not programmed line after line. Instead, they receive feedback on the quality of their solutions and then re-arrange their internal structure by themselves. This is why researchers refer to them as black box systems. The problem is that you can never be entirely sure what exactly a system like this has learnt, and it does not really help us gain a better understanding of natural intelligence.
McGurk effect
The McGurk effect proves that our vision influences what we hear when someone speaks. It is one of the most well-known experiments in perceptual psychology. Participants watch a video of someone speaking the syllables ‘ga-ga’. However, the recording is manipulated so that the sound which is actually played is ‘ba-ba’. The surprising result: 98 percent of test persons claim to hear ‘da-da’. The speech centre in the brain obviously reacts to both acoustic and visual signals and tries to align them.
Many teams across the globe are currently working to find a solution to this problem, with most of them concentrating on image-processing systems. Krauss and his team want to increase the transparency of language-processing systems. The first thing they noticed was the lack of previous data. Researchers focusing on image recognition have huge databases at their disposal. Data on understanding language, however, are hard to come by and tend to have little relation to the real world. Most of the data available is obtained from studies on understanding individual words. Krauss has now filled the gap with his audiobook study, creating the first large dataset taken from a natural setting. He can now use this data to test any number of new hypotheses and perhaps elicit some new insights into how the brain processes language.
One of the experiments the researcher and his team have carried out involved an algorithm for completing ten-word sentences by adding the eleventh word. ‘We wanted to know how the system organises tasks like this. Our experiment showed us that it does not base its answer on the content of the sentences but rather on the part of speech of the missing word. That is not a bad strategy,’ explains Krauss. Perhaps this is a language-processing principle which is shared by the human brain and learning algorithms in spite of all their differences. Bearing this in mind, perhaps going from the human brain to the computer and back again is actually a short cut rather than a diversion: it is easier to do experiments with a computer. But only once the necessary data have been generated – thanks, for example, to participants listening to audiobooks whilst wired up to a magnetoencephalograph.
About the author
Manuela Lenzen works as a freelance science journalist for various large German-language newspapers and magazines. She studied philosophy, history, political science and ethnology.
FAU research magazine friedrich
This article first appeared in our research magazine friedrich. You can order the print issue (only available in German) free of charge at presse@fau.de.